Table of Contents
Need Help? Get in Touch!
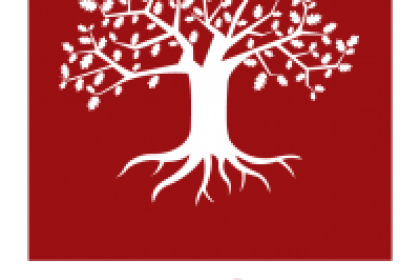
Last Saturday, in what has now been widely publicized and discussed, Uber and Lyft lost an effort, Proposition 1, that would have rolled back a number of regulations on their services. As a result, in one of America’s most forward-thinking tech centers, the services stopped operating almost immediately.
Andrew Watts wrote a great analytical piece on the possible reasons why their effort failed, but what we’d like to do is propose how Uber and Lyft, in future efforts, can use data science as a key part of their strategic arsenal.
They do it everyday as a part of their cutting-edge ride-sharing apps, so why not as part of their campaign outreach?
Model who is likely to vote, but also how
Austin has 509,242 registered voters — most of whom are lower turnout, general-election voters (61% haven’t voted in any of the last four primaries). It should be no surprise, then, that the May 7th election represented a unique challenge for modeling a likely voter electorate: it was a Saturday special-election, during a busy Spring weekend, focused on a single issue proposition.
Not surprisingly, in a town of almost 900k residents, less than 10% voted (88,241).
Following recent trends in Texas — we worked the Houston, TX mayoral race in 2015 as well — early voting represented 62.1% of the total votes (it was also over 50% in Houston’s November election). Not surprising at all, since the last thing most voters want to do on an Austin Saturday is trek to their local polling place.
However, as we look at the press accounts of the campaign, there’s little evidence that the Uber/Lyft effort specifically targeted likely early voting individuals with specific messages or frequency. Traditional AB/EV outreach, like targeting past early voters with a mail piece with their polling location, was probably used but with such a unique election and low turnout likelihood, a deeper look was needed.
Efforts like this, instead of just relying on a traditional universe of likely voters, requires a detailed model, to the voter level, of not only who is likely to vote, but what’s the method they’ll use to vote, and how we can best message to that individual to mobilize them. This must be designed around the specific election scenario at hand to maximize lift.
Modeling likely persuasion targets
To us, one of the most important lines from Andrew Watts’ piece was this:
Uber and Lyft also did hardly anything to convey why voters should vote for Prop 1
Think about that for a moment….in an effort that reportedly cost $8.6 million, the frequency and impact of positive persuasion messages was lost on Austin voters.
Data science and modeling, combined with rich and diverse research on Austin voters, could have allowed them to specifically score and model the likely-voter electorate based on issue impact and saliency.
For example, there is likely a broad segment of voters who are unaware of impact that ride-sharing services like Uber and Lyft have on DUI statistics. Another example would be the impact that Uber/Lyft have on employment numbers and job availability for local Austinites. Many Austin voters simply couldn’t recall these issues being talked about, which is a missed opportunity.
With a data science-driven, targeting-based approach, instead of forgetting the positive messages laid out by their effort altogether, voters would actually hear about the things that they care about, which would impact their voting decision.
Tactical efficiency
One of Andrew’s last impactful points was the saturation of voter contact that many Austinites received. Twitter reports show some voters with mailboxes stuffed with mail and timelines overrun with ads. However, according to Andrew:
They just kept going
There is a better approach. Data science and analysis allows campaigns to not only review what methods are most efficient for reaching different slices of the electorate, but to also measure the effectiveness of that outreach.
Not only does this mean that non-eligible voters (like UT students from out of state) would be avoided (and thus, no money wasted on them), but dollars can be adjusted throughout the campaign to emphasize tactics that are working. In many cases, the fees that we charge a client for our data science consulting services are more than recouped using efficiencies and eliminating waste — something that seems to have been possible in Austin.
There’s no doubt that Uber and Lyft both value and have built their companies on a foundation of data science. In fact, we use some of the same technology at our company to employ modeling and predictive analytics for our political, corporate, finance and other client verticals.
Our goal is also not to Monday-morning quarterback a previous effort. We simply know that using data in such a unique turnout and messaging scenario helps an effort like this be more targeted and more efficient. Lessons we have learned at the Presidential-campaign level can be applied to local efforts like this, thanks to the scalability of data science and technology.
We hope that our suggestions of how those techniques can apply not only to the Uber and Lyft apps, but to their outreach efforts, will impact future campaigns and create a culture of efficient, targeted and measured strategy that will be successful in this modern age.
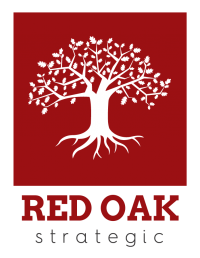
Contact Red Oak Strategic
From cloud migrations to machine learning & AI - maximize your data and analytics capabilities with support from an AWS Advanced Tier consulting partner.
Related Posts
Data
Introduction The age of Big Data is upon us. From smartphones to sensors, the volume of data generated daily is staggering and presents chal...
Patrick Stewart
Data Processing
Introduction The advent of artificial intelligence (AI) in the business world is not just a fleeting trend; it's a transformative force resh...
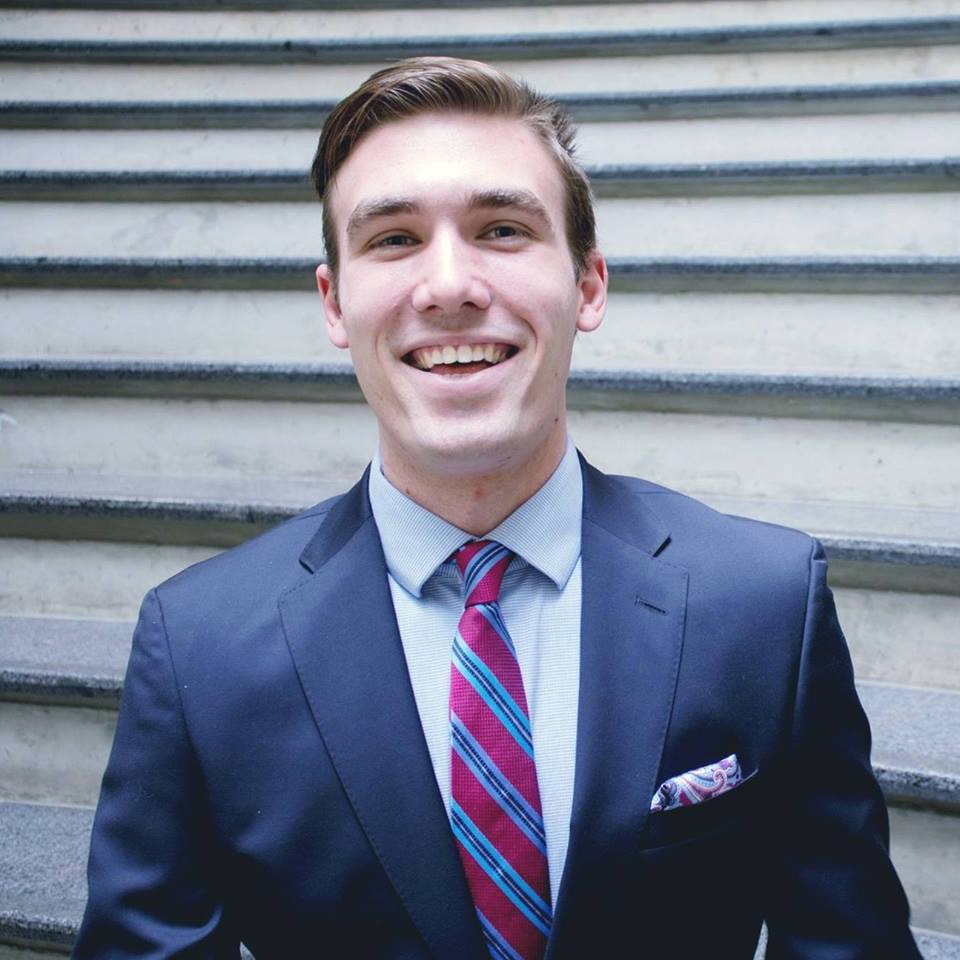
Tyler Sanders
Data
Amazon Web Services (AWS) is, in my opinion, the single most powerful business-building tool in existence today. Bold claim, but with more t...
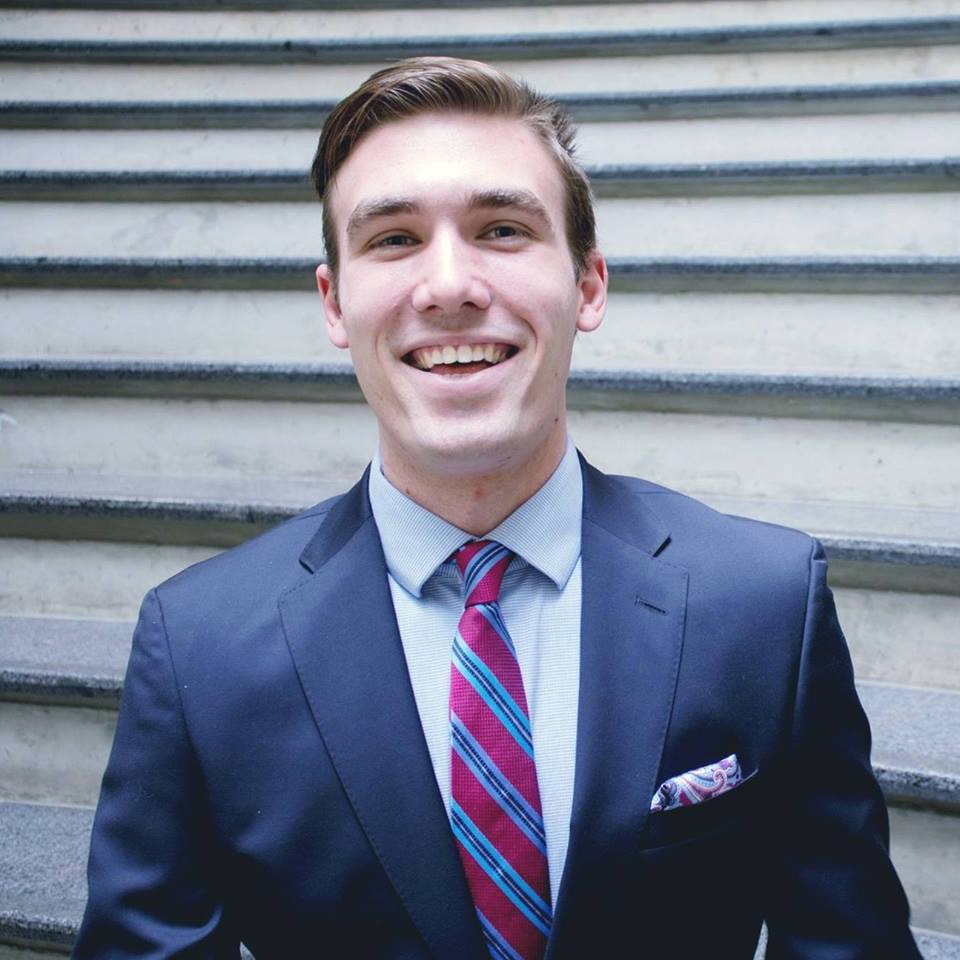
Tyler Sanders
Ready to get started?
Kickstart your cloud and data transformation journey with a complimentary conversation with the Red Oak team.